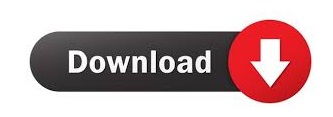
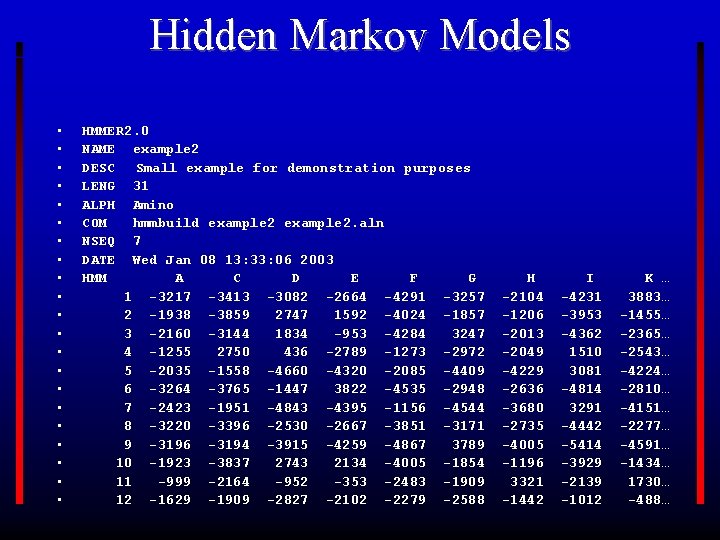
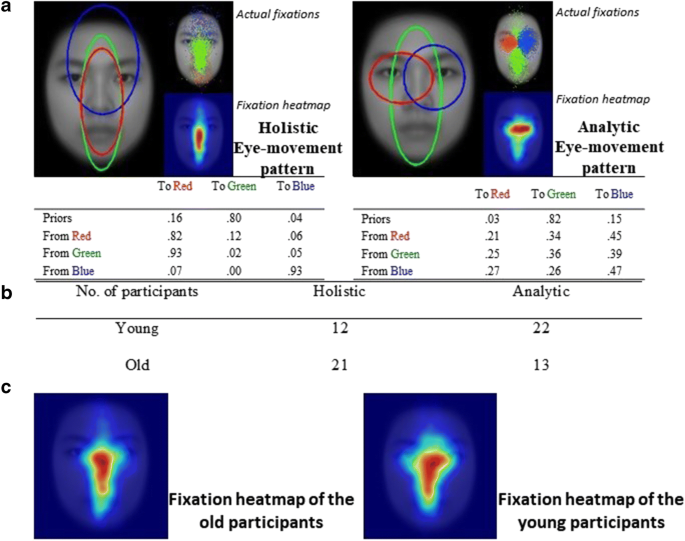
These examples show the ability of the HMM to represent behaviourally relevant dynamics within its states and state time-courses.

This includes time-varying oscillations during finger tapping in source reconstructed MEG signals ( Vidaurre et al., 2016), identifying processing stages during cognitive tasks in EEG ( Borst and Anderson, 2015), and finding state sequences associated with perception and recall of narrative structure in fMRI ( Baldassano et al., 2017). The HMM can be used to flexibly characterize dynamic states across a range of data modalities and has been applied to an increasing number of tasks and datasets. Previous applications of HMMs to source MEG data have shown state switching between large-scale networks on the order of 100 ms ( Baker et al., 2014). The result is that the HMM is capable of identifying dynamic re-organization of whole brain networks on fast, milliseconds time-scales. State-wise functional connectivity can be robustly estimated by pooling data across all visits to each state, though each individual visit may only last for tens or hundreds of milliseconds.

The HMM estimates adaptive state visits or ‘windows’ in a data driven manner. Hidden Markov Models (HMMs) do not require pre-specification of sliding window length, instead the relevant time-scales are learnt directly form the data. In particular, a short window length will give poor estimation of the graphical networks, while a long window length limits the visibility of fast time-scale in the sliding window analysis.Īlternatively, Hidden Markov Modeling can be used to segment observed data into a set of discrete functional states that reoccur over time. Yet the temporal resolution of sliding window approaches is limited, as each window requires relatively large amounts of data, typically several seconds in length. Network dynamics are observable using sliding time-window approaches on both resting state ( de Pasquale et al., 2010, 2012) and task data ( O’Neill et al., 2017b). The signatures of these dynamics may be observable in M/EEG data, although robust modeling of the evolution of functional connectivity on rapid cognitive timescales remains a challenge ( O’Neill et al., 2017a). It is likely that the brain supports complex thought and behavior by dynamic recruitment of whole-brain networks across millisecond time-scales. The analysis pipeline demonstrates a general way in which the HMM can be used to do a statistically valid whole-brain, group-level task analysis on MEG task data, which could be readily adapted to a wide range of task-based studies. We apply this to a freely available MEG dataset in which participants completed a face perception task, and reveal task-dependent HMM states that represent whole-brain dynamic networks transiently bursting at millisecond time scales as cognition unfolds. We show how the HMM can be inferred on continuous, parcellated source-space Magnetoencephalography (MEG) task data in an unsupervised manner, without any knowledge of the task timings. Here, we present one potential solution using Hidden Markov Models (HMMs), which are able to identify brain states characterized by engaging distinct functional networks that reoccur over time. The signatures of this process may be observable in electrophysiological data yet robust modeling of rapidly changing functional network structure on rapid cognitive timescales remains a considerable challenge. 3Department of Experimental Psychology, University of Oxford, Oxford, United KingdomĬomplex thought and behavior arise through dynamic recruitment of large-scale brain networks.2Wellcome Centre for Integrative Neuroimaging, University of Oxford, Oxford, United Kingdom.1Oxford Centre for Human Brain Activity, University of Oxford, Oxford, United Kingdom.Quinn 1,2*, Diego Vidaurre 1,2, Romesh Abeysuriya 1,2, Robert Becker 1,2, Anna C.
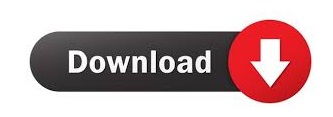